Harnessing data for intelligent planning by tapping into multiple data sources is a must for most enterprises today. Job Market data is a major source that involves extracting job posts and different data points in it. The data points may include skill-sets, salaries, locations, work experience requirements, and tools one needs to be adept with. All this data can shine light on valuable insights. The data can help figure out the financials or health of a company, how a sector is faring in current years, how a region has grown in terms of industrialization, and more. Even stock market or real estate predictions can be performed using real time and historical job data.
However, like any other data, job data too needs to be handled carefully so that companies can stay on the right side of the law and avoid large lawsuits that may leave them bankrupt. Ethical and legal concerns regarding privacy and security must be taken into account before undertaking any major job data scraping related projects. For companies operating in multiple companies the challenge is multi-fold given that every region may have its own law.
Understanding Legal Frameworks
The first step to complying with the law is understanding the law. Laws like GDPR in Europe and CCPA in California law down the framework on how data can be used, stored, transferred, and protected. They also define when and how data may also need to be deleted when required.
You need to ensure there are regular internal data audits to be sure of how your data is being managed, transferred and accessed. That is the only way you can be sure that you are following all the rules.
Ethical Data Collection
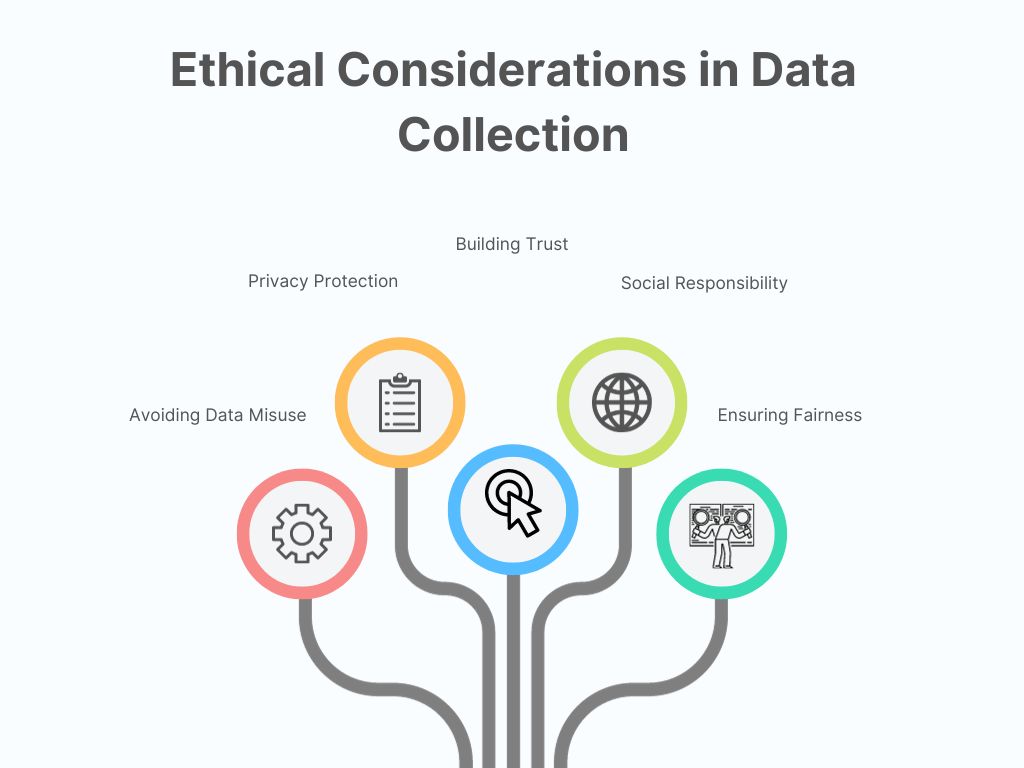
Image Source: Ethics in Data Collection: A Priority for PromptCloud
Apart from following the rules laid down by governments and organizations, you also need to ensure that the data collected is being used ethically and transparently. Job data scraping should not infringe upon the personal or professional privacy of individuals. For instance, in case you are scraping data related to the qualifications and experience of individuals who hold a certain job title, you should not be capturing names or other data points that may expose identities.
For most job data-related applications, we should only be storing and using the data points that we need, and remove the noise. Using less data can help companies implement better control over data access as well. You wouldn’t want to get penalized for having data on your system which you aren’t even using.
Securing Data- Both in Rest and in Transit
Every company needs to have some checks and balances when it comes to using or accessing data. Every data point need not be made accessible to everyone in the company. Only those handling raw data or generating graphs and reports from the data may need access to a large part of the data. Compartmentalization is important so that data doesn’t end up in the wrong hands and to ensure that there is no data leak. Data leaks and exposures can not only lead to legal challenges but also bring down the reputation of a brand.
Data saved in cloud services needs to be encrypted and properly secured via different authentication and authorization protocols so that there’s no unwanted access or data loss. Data in transit also needs to be protected via different methods available so that only the end user can correctly decrypt the data. This way, even if someone can access the data while in transit, they wouldn’t be able to understand it or use it.
Data Anonymization in Job Data Scraping
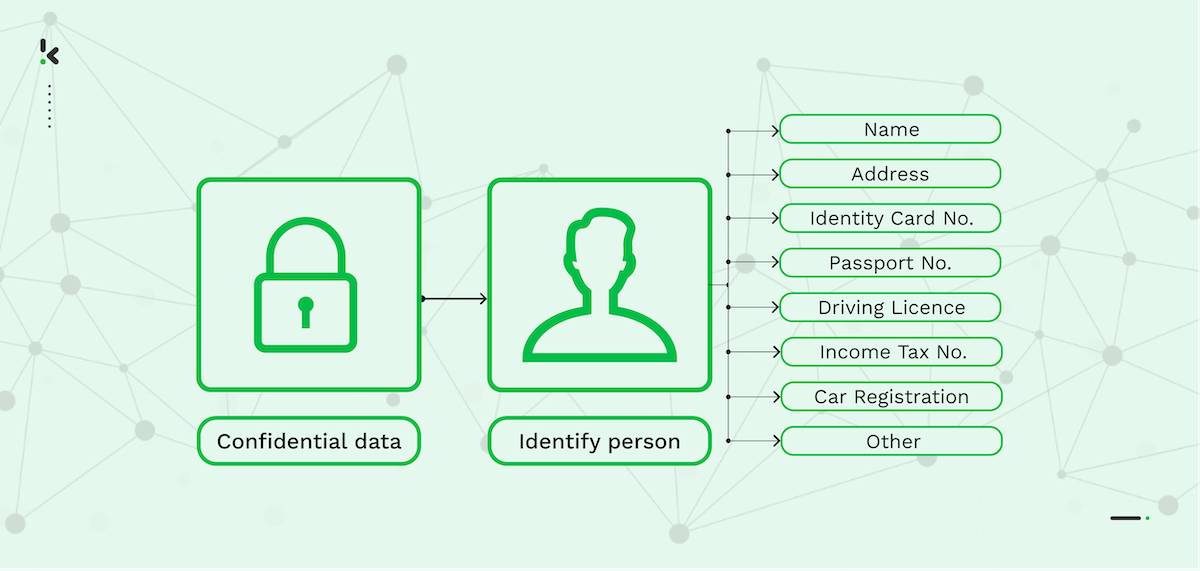
Image Source: What is Data Anonymization? Secure Your Data – Klippa
You may want to anonymize data when using it for purely analytical purposes. For example, if you are calculating the salary bands for different roles, there is no need to have the company name present in that dataset. When handling job data, a lot of identifiable information may creep into your datasets. Any of it that’s not required can be anonymized. In case you still want to identify different entities just to find out metrics like how many job entries were posted by a company, you can choose to replace names with ‘unique ids’.
Transparency and Accountability
How and where you are using the job data is important. This needs to be informed to all stakeholders. Clear communication on privacy policies, user agreements, and updated documentation should be available. A ‘how to reach out to us’ section should also be available in case you are using someone’s data and they want you to remove it.
Establishing processes may seem cumbersome, but once they are in place, they will act as railings to ensure there are no falls. You will also be able to prove your claims if you ever need to, in a court of law.
Impact assessments
Companies managing large volumes of data need to have regular Data Protection Impact Assessments to identify and mitigate the risks related to job data scraping. DPIAs are particularly important when you are laying down new processes or pipelines and need to validate if they are safe and sound.
When it comes to job data scraping, there have been multiple high-profile judgments we have seen in recent years-
- hiQ Labs vs LinkedIn– LinkedIn wanted to stop hiQ Labs from scraping publicly available data from LinkedIn and analyzing workplace issues. It was ruled in favor of hiQ Labs.
- Clearview AI- The company did not directly extract job data but scraped millions of images from over the internet for testing out its facial recognition software. The company was fined in multiple countries.
The distinct difference between the two judgments shows that when data is scraped and used ethically, the law will side with you. However, for companies to tiptoe around the law and figure out what’s right and wrong may be a long, cumbersome, and expensive process.
Instead, using a job data scraping solution like JobsPikr may work wonders. When using a DaaS solution like ours, all you need to worry about is the problem statement that you are trying to solve and how job data will help you in your journey.
You can get the raw job data, by filtering it by region, sector, job titles, and skill sets. You may also want to search for jobs using keyword matching against the job descriptions. In case you want to use dashboards instead of raw data, JobsPikr also offers different Dashboards targeted toward resolving problem statements like Job Comparison, Skillset Matching, and more.